Case Western Reserve researchers pioneer new computer imaging method to detect likelihood of cancer based on changes in blood vessels and regions outside tumor
Scientists in Anant Madabhushi’s computational imaging lab at Case Western Reserve University have started thinking outside the box—or in their case, looking outside the tumor.
They’re hoping that this novel computerized approach represents a historic leap in diagnosing cancer using just routine CAT scans.
If proven successful, it would be a new, non-invasive way to more easily, accurately and inexpensively identify whether certain tumors—especially nodules frequently found on lung CAT scans, for example—are cancerous or harmless.
Currently, lung-cancer screenings involve a radiologist identifying suspicious-looking nodules on a CAT scan. Patients are then subjected to invasive and expensive surgical biopsies or other procedures to analyze the nodules.
So far, however, Case Western Reserve researchers have used a computer to analyze the regions outside the tumor and the blood vessels nearby to successfully predict whether those nodules are cancerous—not through deeper examination or by cutting into them.
Looking outside the tumor
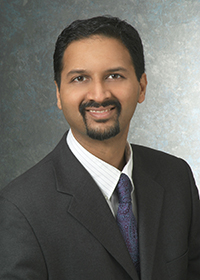
“We’re now doing something that radiologists do not typically tend to do,” said Madabhushi, the F. Alex Nason Professor II of Biomedical Engineering at the Case School of Engineering. “Radiologists have been looking at CAT scans for 40 or 50 years, but they have never looked in these locations or found what we’ve found.”
Madabhushi and his team, which published a paper last month on one aspect of the research, are using “deep-learning” diagnostic computers to examine the shape of blood vessels feeding a nodule found on a lung CAT scan and in regions surrounding the tumor. They’re finding previously unrecorded changes in those blood vessels—or otherwise healthy surrounding tissue—which accurately predict a cancer growth nearby, in this case, lung cancer.
That simple and inexpensive diagnosis from an existing CAT scan could then determine whether a patient needs more invasive and expensive procedures, Madabhushi said.
“A lot of these nodules show up on a scan as ‘indeterminate,’ but 98 percent turn out to be benign, but only after more tests are done,” he said. “So there’s the economics of it, but also the anxiety for the patient.
“We don’t really do a great job of lung-cancer screening because we don’t have better tools. This would be a far, far better tool.”
Madabhushi’s Center for Computational Imaging and Personalized Diagnostics at Case Western Reserve, founded in 2012 and now with some 50 researchers, is a global leader in the detection, diagnosis and characterization of various cancers and other diseases by meshing medical imaging, machine learning and artificial intelligence.
This new research is similar in that it employs both the very simple—either a tissue slide or a CAT scan—and the complex: computers that can quickly and accurately find similarities and minute differences in the images and associate them with cancerous and non-cancerous cells.
Latest research published
The latest research has been highlighted in a pair of recent publications in which Madabhushi was the senior author:
- Mehdi Alilou, a senior research associate in biomedical engineering, was the lead author on a study showing that computer-extracted patterns of “vessel tortuosity”—or twisted blood vessels—in lung nodules could distinguish between malignant and lung nodules with 85 percent accuracy.
- Niha Beig, a PhD candidate in biomedical engineering, was the lead author on a paper published last month in the journal Radiology. The research demonstrated that a combination of computer-extracted patterns of heterogeneity within and outside the tumor could distinguish benign from malignant nodules on CAT scans with 80 percent accuracy—compared to 60 percent accuracy by a radiologist.
“The No. 1 reason I’m excited about these papers is that we are in truly uncharted territory,” Madabhushi said. “We’ve all been trained that ‘the money is in the tumor,’ but what these papers demonstrate unequivocally is that there is a lot of signal outside the tumor, too.”
For more information, contact Mike Scott at mike.scott@case.edu.
This article was originally published Dec. 18, 2018.